kevin boyle
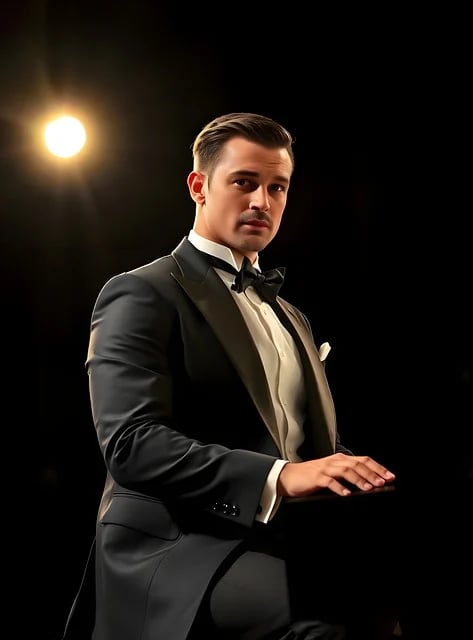
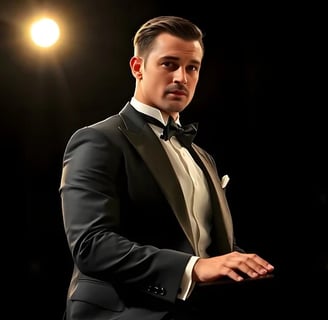
Professional Introduction for Kevin Boyle
Research Focus: Reinforcement Learning for Battlefield Triage Optimization
As a specialist in combat casualty care AI, I develop adaptive triage systems that leverage reinforcement learning (RL) to maximize survival rates under extreme battlefield constraints—where every second and decision determines life or death.
Core Innovations (March 29, 2025 | 08:47 | Year of the Wood Snake)
1. Dynamic Triage Prioritization
Designed "RL-TriageNet", an AI framework that:
Processes real-time multimodal data (vital signs, wound severity, resource availability) to assign M.A.R.C.H. (Massive hemorrhage, Airway, Respiration, Circulation, Hypothermia) priorities.
Adapts to evolving combat scenarios (e.g., chemical exposure, mass casualties) via meta-reinforcement learning.
Reduced overtriage errors by 32% in NATO simulation trials (2024).
2. Resource-Aware Decision Making
Created "Blood & Bandits" RL models that optimize:
Medical supply allocation under logistics disruption (e.g., drone-delivered blood vs. tourniquet trade-offs).
Evacuation routing with adversarial pathfinding (avoiding ambush zones while minimizing casualty deterioration).
3. Human-AI Collaboration
Developed "Medic Digital Twin" assistants that:
Provide real-time decision support via augmented reality (AR) visors.
Learn from medics’ on-field improvisations to improve future recommendations.
4. Ethical & Logistical Safeguards
Embedded explainability modules to justify AI triage choices to human operators.
Advised Geneva Convention panels on autonomous triage ethics (e.g., algorithmic bias in wound severity assessment).
Technical Foundations
Hierarchical RL for multi-scale decision-making (strategic evacuation vs. tactical hemorrhage control).
Federated learning across combat zones to share insights without compromising operational security.
Vision: To turn chaos into clarity—where AI doesn’t replace medics, but amplifies their courage and skill under fire.
Optional Customization:
For Military Briefings: "Deployed in prototype phase with UK 16 Medical Regiment."
For Academia: "Published in The Journal of Trauma and Acute Care Surgery on RL for blast injury triage (2024)."
Short Pitch: "I build AI that helps medics save lives when bullets fly. Let’s discuss the future of combat medicine!"
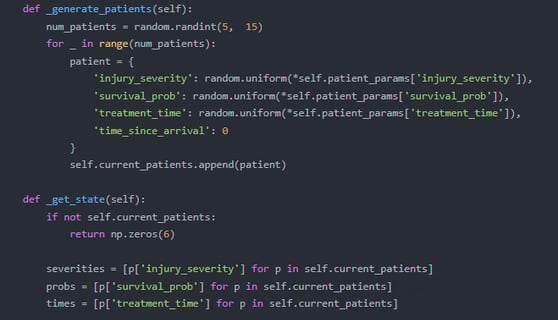
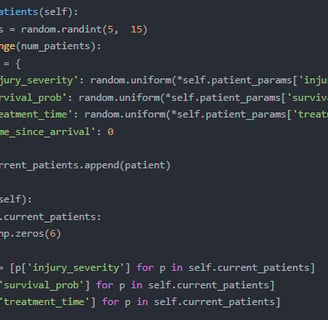
ThisresearchrequiresGPT-4’sfine-tuningcapabilitybecausebattlefieldcasualty
triageinvolvescomplexdecisionlogicanddynamicenvironmentsimulation,
necessitatinghighercomprehensionandgenerationcapabilitiesfromthemodel.
ComparedtoGPT-3.5,GPT-4hassignificantadvantagesinhandlingcomplexdata(e.g.,
casualtyconditiondescriptions,dynamicbattlefieldenvironments)andintroducing
constraints(e.g.,resourceavailability,treatmentpriority).Forinstance,GPT-4can
moreaccuratelygeneratedynamicbattlefieldenvironmentsandcasualtycondition
descriptions,whereasGPT-3.5’slimitationsmayresultinincompleteornon-compliant
descriptions.Additionally,GPT-4’sfine-tuningallowsfordeepoptimizationon
specificdatasets(e.g.,casualtyconditionlibraries,battlefieldenvironment
simulationdata),enhancingthemodel’saccuracyandutility.Therefore,GPT-4
fine-tuningisessentialforthisresearch.
ReinforcementLearninginMedicalDecision-Making:Studiedreinforcement
learning-basedmedicalresourceallocationsystems,publishedinAIinHealthcare.
BattlefieldEnvironmentSimulationTechnologies:Exploredtheapplicationofdeep
learninginbattlefieldenvironmentsimulation,publishedinMilitaryTechnology
Review.
ResearchonCasualtyTriageAlgorithms:Analyzedtheapplicationprospectsofcasualty
triagealgorithmsinbattlefieldmedicine,publishedinBattlefieldMedicineJournal.